What Gets Mismeasured Gets Mismanaged
Marketing is an investment. An investment in potential new clients or customers generating revenue. Unfortunately, the road to acquiring new clients has many potholes, blind curves, oil slicks and bandits. Configuring and continuous monitoring your campaigns well will avoid the usual dangers, except the most dangerous one: the bandits aka fraudsters in the digital world. To address ad-fraud, click fraud, lead gen fraud sucessfully you’ll need specialistic tools which again have to be updated continuously in order to keep up with the evolving threats.
The one million dollar question is ‘what is successfully’ when addressing these types of fraud?
Flagging 100% of all visitors as fraud will definitely solve any fraud problem. But, you don’t have any business left. Flagging only 1% of the visitors will just catch low hanging fraud. Unfortunately, catching simple fraud doesn’t stop compliance issues, and in case you're generating leads flagging simple fraud won’t stop your legal department receiving a ton of TCPA demand letters [6]. Both scenarios are far from ideal, but what is?
The ideal situation is when fraud is flagged as fraud and humans aren’t! Simple as that. Except, that’s not what the average fraud detection vendor delivers. This means on one side the trade-off of a fraud detection not flagging fraud is the financial damage of the unflagged fraud. On the other side the trade-off of a fraud detection flagging too much is the financial damage of missed business. The question is: What is worse? Accept some fraud or accept some missed business, and how much is acceptable?
Legacy ad-fraud detection
Figure 1 shows how legacy fraud detection separates human visitors from the fraudulent visitors. This is based on their ~1% fraud reported quarterly. We all know fraud is more prevalent, though the reported percentage remains ~1%. Using 12% of fraud as the ‘true percentage of fraud’ this means their detection misses 11/12th of the fraud. That looks bad, but the real question is: How costly is this, financially from a business perspective?
Trigger happy ad-fraud detection
Abraham Kaplan called it “the law of the instrument” and it may be formulated as follows: “Give a small boy a hammer, and he will find that everything he encounters needs pounding.” [1] . The same behavior can be seen in fraud detection solutions too eager to find and flag fraud, anything out of the comfort zone (eg. browsers with an Asian language), anything out of the ordinary (eg. custom fonts installed), anything deviating from the default setting (eg. other javascripts on the website override certain browser functionality and/or polyfills [2]) affecting the detection, will be considered fraud.
This causes fraud detection solutions to flag a much higher percentage of fraud compared to the true fraud percentage. These incorrect flagged visitors are false positives. This means a portion of your human audience will be ignored by these trigger happy fraud detection vendors. Ignoring humans will cost you business and will affect your sales numbers.
Figure 2 shows how trigger happy fraud detection has a high false positives rate. The main reason is that these fraud detection solutions use “soft metrics” to flag fraud. Without having a groundtruth a baseline cannot be established and thus you’re just guessing who is a normal human visitor and what isn’t. As we all know “Assumpsion is the mother of all f-ups”, then why do these vendors flag like this? Here, I’m assuming it’s because of enshittification [3], hubris, and self flattery :-).
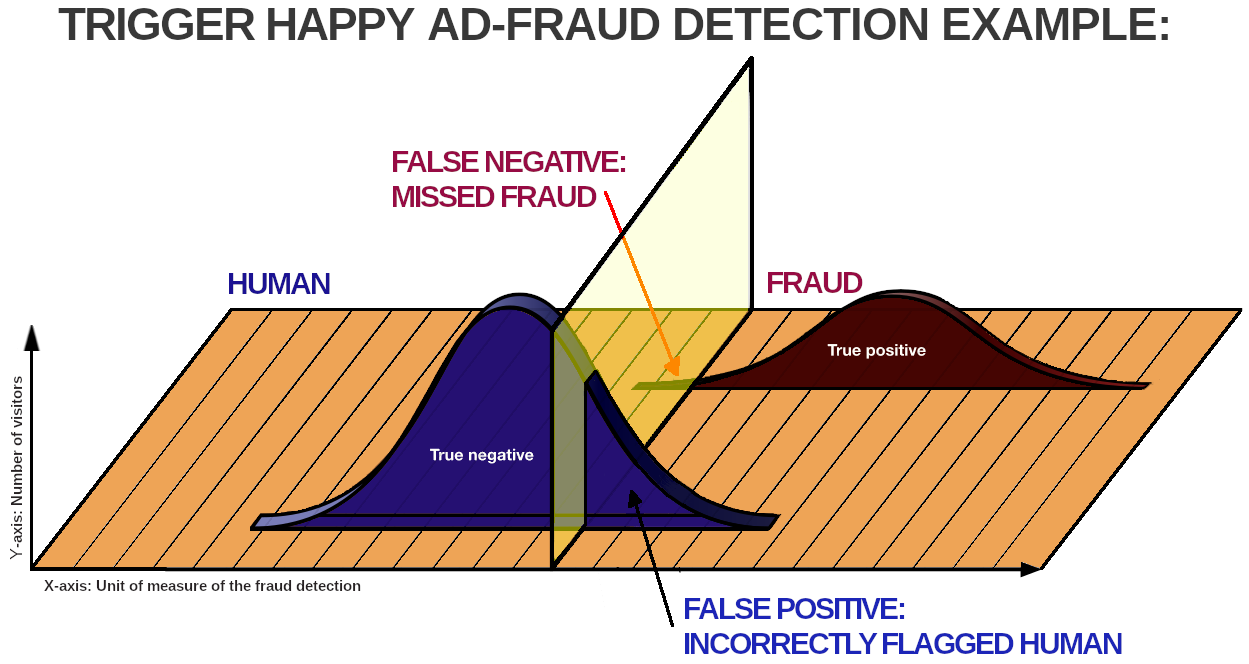
But, back to business. A high number of false positives sounds really bad, but again the real question is: How costly is this, financially from a business’ perspective?
Business impact
In order to model the business impact an Excel document has been created. This contains all the parameters and metrics of a typical digital marketing campaign. As campaigns differ over time, per vertical and per country, state, city, the sheet enables you to configure price (CPM), click-through-rate (CTR), conversion to sale, customer lifetime value (CLV), ad-fraud detection costs, and how much ad-fraud is detected. Based on these parameters it calculates the cost per click (CPC), customer acquisition costs (CAC), the CLV:CAC ratio, the false negative rate, and the false positive rate.
Figure 3 shows the comparison between a legacy vendor (1% fraud) and a trigger happy vendor (30% fraud). Both fraud detection solutions are equal priced and the model doesn’t take include any refunds or credit traffic based on the fraud percentage. On the other side it also doesn’t include the business costs of poor data quality, and potential litigation risks. The model shows that missed business (18% FP) affects the ROI performance of your campaign(s) much more than the wasted dollars on fraudulent impressions and/or clicks (11% FN) . That makes perfectly sense, simply because any business has to outweigh the campaign investment.
But, I can hear you thinking, what if the legacy fraud detection vendor is much more expensive? Let’s triple the costs and recalculate the campaign using an ad-fraud detection cost of 0.0006 per verification. This will affect the pricing, and increases the CAC from $62 to $66 and respectively lowers the CLV:CAC ratio from 4.52 to 4.24, as can be seen in Figure 4. In this scenario, pricewise, flagging only 1% of the fraud and thus missing 11%, is still 14% cheaper than flagging 18% too much.
In order to compare different fraud levels a lookup table has been constructed. The horizontal x-axis contains the true fraud percentage. As you may have read in the Oxford Biochronometrics ’ affiliate benchmark report [5]: In normal circumstances the fraud averages out at 12%, but may differ greatly per source. The first step is to look up the fraud percentage of a source (eg. Facebook, Bing, etc.) in the benchmark report, and write down the percentage. The second step is to look up what percentage your current fraud detection vendor flags and reports. Now you have two percentages: On the x-axis go to the column using the percentage of step 1, and subsequently go to the row using the percentage of step 2. This cell contains the performance value as a CLV:CAC ratio based on your true and vendor reported percentage combination. The table only contains percentages below 40%. The table has been generated using the exact same logic and ad-verification pricing as shown in Figure 4.
Based on the table it can be seen that having a vendor reporting a ~1% fraud percentage is (financially) better for the ROI of your marketing campaign than having a trigger happy fraud detection overreporting and make you ignore human traffic. Of course when refunds,credit traffic, data poisoning, (TCPA) [6] litigation risks are added to the mix these results will change slightly. In the end: The best scenario is to flag and report fraud accurately, where the reported fraud matches the real fraud, without FPs and without FNs. Okay, granted, digital marketing campaigns without any fraud would be even better, but we all know that’s wishful thinking.
How does Oxford BioChronometrics know the real fraud percentage?
Oxford Biochronometrics has been founded by individuals NOT having a background in digital marketing. Our background has been in cybersecurity, designing and building a real-time solution to detect and flag fraudulent transactions in Internet banking sessions. The experience of designing, building and embedding a real-time fraud detection product into a large bank with all its regulations, compliance, etc. has set the standard for creating the Oxford Biochronometrics fraud products. Yes, we had to learn how digital marketing in general works, how the ecosystem, the attribution, pixel tracking, viewability, calculating the ROI, ROAS, etc. works. Most of these are based on technical solutions mapped to a marketing functionality, financial instruments, or similar to stock markets and stock exchanges, which we were already familiar with.
“Keep your friends close, but your enemies closer” -- the Godfather part II [3]. As a company you would like to know what your competitors are doing. This is why every now and then you’ll take a look at your competitors’ websites, blogs, results, etc. and one of the most interesting freely available piece of information is their public part of the fraud detection software: The JavaScript collecting data from the browser. Anybody will be able to download this, read the code and based on that be able to understand what data points the JavaScript code collects and conveys. If you have a programming and cyber background this is a fairly simple exercise, mentally similar as baking an apple pie! Although some companies try to protect their JavaScript and try to make your life miserable by obfuscating and encrypting the JavaScript code, with the proper tools and knowledge that can be reversed, and then it’s still doable [4].
Real results is what counts. Having good feedback from your clients, “your detection saves us a ton of money and provides us clean data”, is nice. But, firsthand seeing how one of your clients grows from a startup to a scaleup and then ~18 months later they are being bought for multiple billion dollars, while their #1 revenue comes from digital marketing on the Internet to get customers to acquire their products. That’s gold and has been the ultimate confirmation that Oxford Biochronometrics has been able to protect their business, provide their data analytics and ML teams with clean data to work with and mitigate litigation risks by filtering out fraudulent leads. This is the long term feedback loop that shows that we consistently keep on performing over a longer period of time.
Combining our cyber background, our freshly acquired marketing knowledge, competitor intelligence and confirmation that we are right on track enables us to know what works and what doesn’t and also what will never ever work. This allows us to understand which fraud detection vendor collects good data and runs good tests in the browser, versus vendors having essential code with design flaws (due to incompetence), ‘soft metrics’ which are interpretable and thus prone to false positives, and the lack of cyber security knowledge to protect their collection mechanism. When you mismeasure, ie. the noise is louder than the signal, you simply can’t distinguish fraud from humans. Any usage of AI or advanced machine learning will not help against that, as the collected data is processed unsupervised and the volumes are just too big to flag it manually. This means an arbitrary line is drawn (the conservative or trigger happy line) to cluster the data into human and fraud clusters. Mismeasured results in a mismanaged outcome: Drawing incorrect conclusions will seriously affect the growth and thus the performance of your business in the long run.
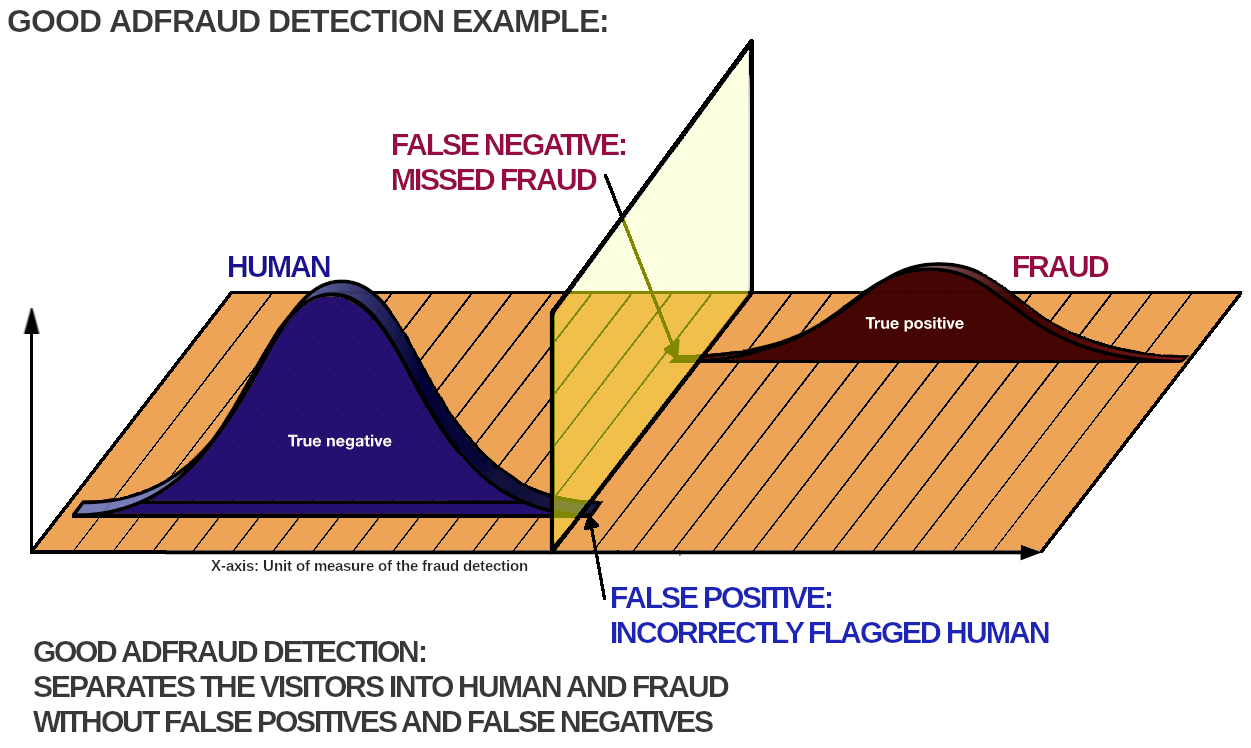
Final words
The costs of doing real fraud detection are high when you need large neural nets and/or machine learning models to separate human from fraudulent traffic. It is not just detection in real-time, but these models needs also to be updated continuously to include new fraud types, new modus operandi of fraudsters, etc. Simply because a fraud detection vendor you don’t want any time gaps between the appearance of a new type of fraud and the detection of that fraud.
Performing real-time fraud detection is a complex process, and hard to do it right. This is much more complex than writing a JavaScript collecting data from the browser. When this JavaScript contains elementary flaws, it sets the standard for their overall detection quality. We can’t see or know how the back-end processes work and how well that software is written and configured, but based on the public available JavaScript code I don’t expect it will suddenly be super-sophisticated high-tech, on the contrary I’m afraid it just isn’t.
10 years ago, in 2013, separating fraud from human traffic was a relatively simple problem. You could just look at the user agent, look at the existence of variables and objects in the browser, webdriver flag, look at some webdriver residues, or rely on a pixel being fired, etc. These days it is much harder and only few understand this entirely throughout the tech stack. Some vendors take advantage of this and sell whatever you want to hear (good marketing, poor product), and unfortunately it is too complex and too technical and thus too time consuming for most companies to verify what really is needed and what happens at their digital frontdoor. Back to the one million dollar question ‘What is successfully in addressing these types of fraud?‘ Answer: Overreporting (ie. higher fraud percentages) isn’t better, underreporting (ie. almost no fraud) is also sub-optimal. Ad-fraud detection has to be accurate. Period!
2023-10-31
Would like the excel sheet? Want to know more? Leave a comment, connect or DM
[1] https://en.wikipedia.org/wiki/Law_of_the_instrument#Kaplan
[2] https://en.wikipedia.org/wiki/Polyfill_(programming)
[3]
[5] https://oxford-biochron.com/affiliate-performance-report/
[6] https://en.wikipedia.org/wiki/Telephone_Consumer_Protection_Act_of_1991